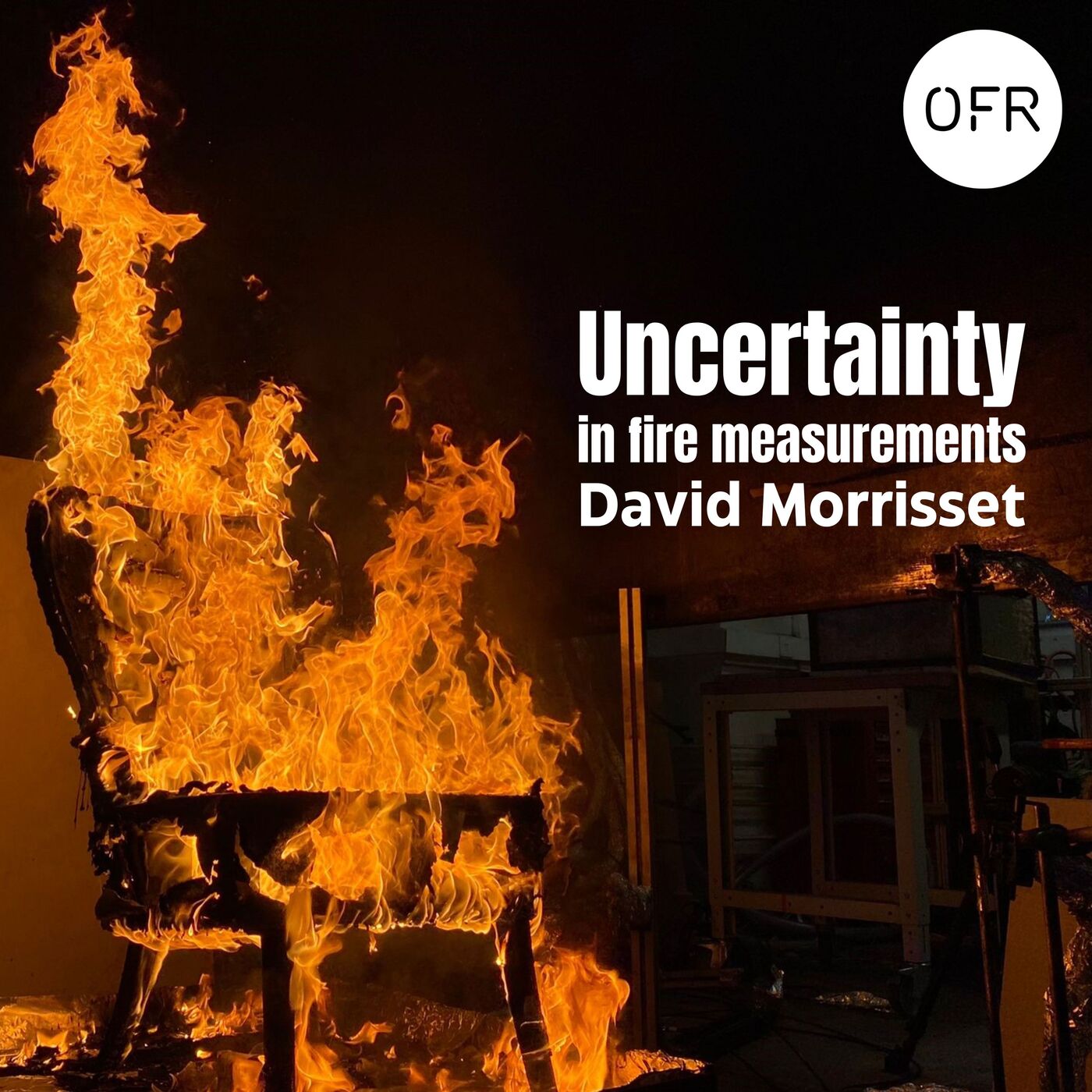
If the word 'uncertainty' sounds extremely boring to you, this episode will prove you wrong. I have invited David Morrisset from the University of Edinburgh to discuss his research on the subject. Whereas in fact David is establishing standard deviations, means and other statistical means of quantifying uncertainty in core fire measurements, the really impactful and important part of his research is on explaining WHY those uncertainties are there. Through physical explanation of processes happening in fire we may grasp a really good understanding why two HRR-time curves of the same object burned in the same lab, in the same way may be so vastly different.
These findings are fundamental for practical fire engineering. The establishment of design fires and their relation to the experiments is discussed in depth. We also talk about how we could establish better design fires for future engineering practice.
Some excellent further reading:
Hello everybody, welcome to the fire science show Today. I am excited. Well, I'm always excited for fire science, but today I am double or triple excited because we're going to go as fire science ish as fire science can get. I have invited a guest PhD student from University of Edinburgh. David Morissette, is about to submit his PhD thesis. Good luck, david. And David has researched a very interesting subject, that is, the uncertainty in the measurements of fire. Not that many people try to touch this difficult and challenging subject, and it's not just developing estimations of how uncertain the measurements of the most basic parameters, like time to ignition or heat release rate are. It's not about finding what's the standard, deviation and average, etc. It's about understanding where do those deviations come in from and what do they mean for fire engineering. This is an extremely interesting topic because it touches the fabric of what we are doing as fire safety engineers. From this episode you will gain so much understanding about what a design fire is, what's a fire experiment and how does the fire experiment translate into a design fire, what goes into it and why those fires come in phases and actually taking into account the physical phenomena and phase transitions that happen during fires. Why is that critical for our understanding of fires and being able to model them. In this episode, we'll go from David's first experiments on PMMA into his extremely interesting experiment series on upholstered furniture and overall those cases. We will discuss uncertainty fires how do they grow, how do they develop, what's happening during the fire and how do we quantify that. I am sure this will be a gold mine for practitioners, especially those who are dealing with modeling fires. So let's not hype this anymore. Let's let's spin the intro and jump straight into the episode. Welcome to the fireside show. My name is Vojci Wimczynski and I will be your host. This podcast is brought to you in collaboration with OFR consultants. Ofr is the UK's leading fire risk consultancy. Its globally established team has developed a reputation for preeminent fire engineering expertise, with colleagues working across the world to help protect people, property and environment. Established in the UK in 2016 as a startup business of two highly experienced fire engineering consultants, the business has grown phenomenally in just seven years, with offices across the country in seven locations, from Edinburgh to Bath, and now employing more than a hundred professionals. Colleagues are on a mission to continually explore the challenges that fire creates for clients and society, applying the best research, experience and diligence for effective, tailored fire safety solutions. In 2024, ofr will grow its team once more and is always keen to hear from industry professionals. Who would like to collaborate on fire safety futures this year, get in touch at OFRconsultantscom. Hello everybody, welcome to the fireside show. I'm here today with David Morissette from the University of Edinburgh. Hey, david, good to have you. Thanks for having me. Man, I'm a big fan. Thanks for coming to the podcast. You're touching some of my most favorite things in the fire science, which is the studies on flammability, design fires and burning grill items. So I cannot wait to have this conversation. But first I need to ask you where this all have started. So when did you figure out that going so deep into the studies on how things burn is the pathway for you in the fire science?
Speaker 2:Absolutely Well. Thanks again for having me. I'm a longtime listener of the show, so it's really exciting to be here with you today. So you're doing great work with this. Thanks, man, anyway. So, as you said, we've been doing a little bit of work, sort of looking at this idea of what is a statistical variation, what is statistical uncertainty. We're looking at fire science, right. So I'm a bit of an experimentalist, sort of that's what I do with my PhD studies at the University of Edinburgh, and so I guess, before we start talking about what is this variation and how many trials do you have to do, I think an interesting question to start with is why do we do repeat trials? So this idea of you know, if I do an experiment, usually don't just do it once.
Speaker 1:Right, or actually in fire science we're not really doing repeat trials. Why should we do repeat trials and how important that is actually?
Speaker 2:Sure, but I mean, if you read any, especially in the flammability literature, if you read experiments on cone calorimetry, or if you read standardized testing procedures, there's a specified amount of repeats, right. But this idea of doing repeats actually comes down to a simple idea, right, the reason we do repeats is this idea of capturing statistical variation, right. So that's that's the mechanism by which we do that. We just specify a certain amount of repeats. So for most of the procedures we'll generally take a few trials and we'll usually take an average of those results and then we hope that those trials that we've captured actually sort of capture the statistical variation that you might expect for that experiment Effectively. It's a consequence of, just like, fear of the unknown. We're hoping that we didn't just get a one off sleep, and so that's the whole idea of doing repeats. But if we just take you know n number of trials, that's an implicit strategy for sort of mitigating your statistical variation. So things that we were trying to look into is how can we do this explicitly and like, very intentionally say, I have a target uncertainty of blank, right and I want to hit that so that I can then report that and so people who use my data know that. This was the level of certainty I have in my data. It's a very powerful sort of tool. But anyway, like you said, there's some studies on that. But let's talk, I guess, a little bit about the origin story of that. So I did my undergrad in California. I was a mechanical engineering student, cal Poly, san Luis Obispo, and we took this one lab course that I'll always remember, because they handed you a box of like 100 resistors you know the electrical components and they asked you to take resistance measurements of 100 resistors. And then we did some very rudimentary statistics on it, sort of look like the main, what's your confidence intervals and things of that sort. Now, obviously it was like a second year undergrad. That was pretty boring in my mind at the time. But what's really interesting is something that kind of stuck with me is this idea that I could take a resistor out of this box, right, and by the end of this exercise I could say with 95% confidence I can tell you where this falls in the range of your mean, plus or minus a certain value. And then I started doing some research at Cal Poly with Rick Emberley and so he's still a professor at Cal Poly and as part of my master's thesis research, I was looking at ignition of PMMA in the cone calorimeter, and so one question I started sort of dabbling with is OK, how many trials should I do? So I opened up the ISO standard, the ASTM standard, and so I used to do three trials or whatever Three, ok, so that's sort of what the standard procedure is. And so I was just cracking all of my experiments and I would say get lunch with some of my friends that were doing biomedical research or something like that, and we'd be talking about our experiments and someone would say, oh so what's your sample size, right? And for your study? Oh yeah, we're doing three, repeats Three. And you would always feel, and they start like laughing hysterically, right, because they're running like hundreds of experiments for a single condition.
Speaker 1:I mean, in the world of real science, like three must be a really silly number Because, like, if you just went with, like oh, I just won, then the reason would be he doesn't care about uncertainty at all. But if you tell those people you're doing three, it's like you're kind of trying, but if you're not really there he's happy. Right, I can imagine it must be funny. But but calm, pmma is something reputable, right?
Speaker 2:I mean, sure, right, but in terms of when we say, like, what is repeatable, like how long is a piece of string? Right, I mean it's a question that depends on the context, right? So yeah, so I mean I'm doing three trials, but the more I talk to these people, I sort of just had that sort of ringing question in my head. Going back to those like resistors, right, and this idea of like, well, at what point do I have enough data? How many trials is enough? Right? So that stuck with me for a while. And when? Something I should say too is obviously because the Coen Calorameters been around for a while. There's been extensive studies on repeatability and reproducibility, and so obviously there is some degree of a basis for which we have an idea of how repeatable certain experiments are. But what I couldn't find is I couldn't find a study that just did a huge number of trials for a single case to just sort of explore the idea of what happens if you have this big data set. And so, if you fast forward a little bit more back in 2020, I had the chance to go to the University of Edinburgh, so I was just visiting to do some experiments while during my masters at the time, and so I got to meet my now PhD supervisors, angus Law and Rory Haddon. And while we were there, we were just in the pub one evening after working in the lab and we were just chatting about doing fire experiments and this idea is like how many trials should I do? Came up and so we started talking and I was asking so, how many trials do you think would be enough? And we got to this question that said well, what if you had 100 data points for something really simple like black PMA in the Coen Calorameter, right? And so then we're chatting around the table and like, oh, that's reasonable, let's give that a try. And what was funny too is across from me was Rory, who I know has been on the show a few times Right. And so I was like you know well, 100 trials. Coen's pretty straightforward, I bet you can smash that in, maybe about a week, you know. And so he kind of gave me a little laugh. He was like he basically made a little bet that I couldn't do 100 good trials in a week. So then what did I have to do? I had to go in on Monday morning and with 100 slabs of PMA.
Speaker 1:But your students. He cannot do it. It's like the most brilliant supervisor strategy I've heard about. I'm going to implement that.
Speaker 2:Anyway. So yeah, absolutely, but yeah. So we ended up with the first 100 data sets, 100 data points, just black PMA in the Coen Calorameter, and we were looking at time to ignition, mass loss rate, heat release rate, sort of all the different data that you get out of the cone, because it's get quite a bit of data out of it and for people that are familiar with that kind of experiment, it's it is straightforward, but you can actually there's a lot you can actually discern from that information. And so once we looked at the 100 data points, we started knowing some interesting trends and so we thought we'd expand a little bit more, and so we ended up doing 100 experiments at three different heat fluxes 20, 40, 60 kilowatts per meter squared and this turned out into an interesting little research project that we published paper on and Fire State Journal.
Speaker 1:I'll link to the paper in the show notes. However, it's kind of interesting I'm looking at the scatterplot of the time to ignition that. You've gotten the histograms you got, and you know one, one thing that hits me, that strikes me that you've said that normally you'd use like three samples, like you just go with three. Let's say you're your sample one to three and you get some value. They're not, those dots are not that far apart, but there are quite some outliers. There's quite a scatter, maybe not a massive scatter, it's not like the things that are all over the place. They go into some sort of natural distribution more or less. But I can imagine picking three of those you know and having completely different final outcome than three others.
Speaker 2:And that's a good observation, right? Because even for our quintessential black PMMA in the cone calorimeter, how quintessential fire science can you get? If you look at the time to ignition for 20 kilowatts per meter squared, if you look at the actual peak-to-peak fluctuations, you're getting upwards of 20% error. So if you'd heard three data points any three random data points, there was a chance however small actually if you run the numbers it's not as small as you think you could get those three points that are off by 20%, right, which throws your mean value off by quite a bit. And so this idea of there is a possibility, if you're only limiting yourself to three, that you could be off from the true mean. And this has implications to all sorts of things, right? Because sure, for engineers listening to the podcast, time to ignition of PMMA in the cone calorimeter isn't necessarily something that is going to propagate itself into engineering design, but what might is things like the parameters that we calculate. You know what is the critical heat flux, what is the thermal inertia of these materials, and a lot of these things are obviously based on these experiments, right? So if we don't account for this potential fluctuations, then how do we account for that when we start propagating that into our parameters.
Speaker 1:Going like even more fundamental how uniform were the properties of the PMMA over the samples that you had? Was this the same slab that you've cut into smaller pieces, like the same density, same thermal bulk and everything?
Speaker 2:Absolutely so. We ordered it from a single continuous sheet of PMMA, right. So that was our attempt to sort of get as repeatable in terms of the properties as you could get. But now that all starts to go out the window if you start looking at things like timber right or any other sort of materials where you have inhomogenous properties or other sort of inconsistencies. It was actually a really interesting master's thesis that just came out of Cal Poly by a student named Jacob David who looked at basically the same sort of things, looking at hundreds of cone tests, but with timber right, and so that's something we're trying to look at right now. Yeah, so we're going sort of back to our main data set on PMMA. So these fluctuations that you see in, like the 20 kilowatt per meter squared case, for example, could be upwards of 20%, right. But as you start increasing heat flux, right, you start seeing for time to ignition. Obviously your nominal value of your time to ignition goes down, but so does your fluctuations. So, based on the fact that there might be those three outliers for the 20 case, and so maybe three isn't enough, right, but all of a sudden, when you go up to say 40 or 60, now the fluctuations are going down quite a bit. Maybe you get to a point where maybe three, four, five trials, maybe that's, maybe that is enough. And so we got to this idea of this. So we got to this question. That was basically how do we discern a threshold? Because I mean, a funny thing that people come up to me at conferences and stuff and they'll be like, so do you think we need to run 100 experiments, sort of like. You know what I'm trying to do and I hope that no one reads the paper and I hope that's not the outcome that people think I'm reading it, because I mean we created the data sets so that we can run sort of the analysis on it. But the reality is is you don't need to necessarily do 100 experiments. But the point is not the number, right? The point goes back to sort of that original question I sort of asked in the intro and it's like why do we do repeats, right? We get back to this point that we want to capture statistical variation, we want to know our confidence in our data set, and so because of that we started playing around with this idea of optimizing your uncertainty, and so you can read the papers for a little more detail, but we basically took this formulation of what we considered the distance from your sort of mere true mean value to your what we'll call our 95% confidence interval, and that scatter be referred to as our statistical uncertainty. Basically, with the data set that I have captured, how well am I capturing the true mean of sort of my data behavior? And so this behavior follows what we would say is a one over the square root of N behavior, so N being the number of trials. So as I collect more data, it's a nonlinear process If we think of that progression instead as sort of the what we'll call the marginal gain uncertainty. So if you take sort of a derivative respect to N for every additional trial and taking how much certainty am I getting back, you can take that function, you can kind of optimize it. What you can do is if you plot that function, we start to realize is going from, say, three to five trials brings you so much more certainty, basically any case that you're looking at, but to go from five to 10 will give you less.
Speaker 1:So the jump from one to three is already a massive one. So I would say that the reason for having three is already. There's a good reason for having at least three repeats, but again adding repeats as more. It's not that every repeat has the same as the previous ones. At some point the retinas start to diminish, right.
Speaker 2:Absolutely so. Exactly, there's this sort of law of diminishing returns. So there's a certain point at which you're no longer, because the reality is is we have to optimize our finite time and resources. For every experiment I'm doing, I'm dedicating resources to doing that that could have gone to a new experimental configuration. So, with this idea of sort of what's our marginal gain, we started seeing that for most scenarios, you bottom that out 10 to 15 trials, and the last thing I want is to start claiming a new magic number, right, Because that changes obviously, depending on your scenarios. But somewhere between three going up to about 15 is where you start to optimize these balance of your variation and this addition of how much certainty am I getting back with each additional trial?
Speaker 1:Well, with the higher hit flux, those returns are less and less valuable. Perhaps, if you calculate it by person, they're still there, but in terms of the value that you're measuring, the returns are smaller and smaller. Now another question that I have is that we put some kind of artificial ways of measuring this. I think we love to measure fires as a function of time. It's kind of artificial because that's a process. It's not a thing that you can put on a time scale and measure how much time is set. It's a process. It has to develop. It's not something that is an explicit property of this material, the same way that we'd like hit release rate. It's also an artificial construct. The mass loss rate, it's an outcome of a process. I wonder how do those different measures fit into this idea of uncertainty? Can we even be certain of hit release rate, or is it inherently uncertain?
Speaker 2:That's a great question, because right now we're keeping things in a very small box talking about this PMMA study, because we're taking single metric of time to ignition. Time to ignition.
Speaker 1:I mean time to ignition. I love it. It's perhaps the least float. Perhaps the least float of them all would be the total hit release. If you burn the sample completely to zero, you should get the least uncertainty out of that because all your material has reacted and your variability is within the efficiency of combustion to some extent. So time to ignition is also something that to me, talks like okay. This is a material that starts in ambient quiescent conditions Possibly, and is subject to a very specified, steady heat flux and eventually the processes in the material cause it to ignite. For me it's like okay, the material starts in the same spot. We always start from the same zero, same ambient, same heat flux. So the process in the material should be the same each time, more or less the same each time.
Speaker 2:I absolutely agree with you, and something that I think we can move to was the next work package we did with this idea. This is cool, everything on the comb scale is interesting, but the exact thing that you're talking about is things like heat release rate. We're looking at time-resolved information. How do we apply these kinds of ideas to that? Because these are the kinds of data that are very familiar with practicing engineers. This is what engineers need. This is what engineers need. If you're developing a design fire, if you're putting this into your FDS model, into your sprinkler calcs or whatever your ceiling jet correlations, you need a heat release input and you need to understand what are the magnitudes, what are your transient aspects of your curve and all those things. So, absolutely so, the next logical step was to scale this up to a complex seal package, and so let's come back to that idea of total heat release later. Remind me to come back to that, because that's an interesting point that comes into play later. Okay, let's start by talking about, well, the cliffhanger oh, I know Keeping it on your toes, but time results. Heat release rate is obviously a natural place to start, and so, when you think of a fuel package that you want to like? First thing comes to mind what do you think of?
Speaker 1:I see an upholstery. You see an upholstery, exactly, yeah, it's like a couch. A couch, armchair, mist armchair. That's what we have in buildings mostly.
Speaker 2:So exactly. So when I was thinking about this, the first thing that came to mind was upholstery couches, upholstery chairs. So I wanted to go as close to that as possible, and so what we ended up doing is I applied for an SFP Foundation research grant one of the student research grants and they were generous enough to contribute to the project, and so I went and procured basically 50 identical upholstered chairs. So the idea was I wanted to get as close to the real thing as possible. I wanted an actual upholstered chair and this is sort of your typical one-seater no armrests, polyurethane foam with a wood frame. That's the kind of deal. So I had an interesting discussion before we did this about what you referred to. There is the NIST armchair. So NIST has this really slick setup where they have the steel frame for an upholstered chair and they can still it with their own homemade materials, highly controlled materials, and so listeners haven't read their research. That's really interesting stuff. On upholstered furniture fires.
Speaker 1:And there's a good podcast episode on the NIST colorimetry database. You should definitely go check that out after this one. There you go, exactly.
Speaker 2:And while that was an idea and I think what they've done with that is excellent we really wanted to sort of go to the ultimate ideas of not realism, because what is real when it comes to a design fire. But we wanted to increase the complexity and actually take a manufactured upholstered chair, anyway. So that's what we ended up doing, and so our core sort of data set was 25 repeats of this upholstered chair in our furniture colorimeter under the same conditions, and we ran it 25 times under those identical conditions. And the idea was to start looking at the variability. And so our ignition source was we had this upholstered chair. We had just a small Bunsen burner, because there's a million different ways you can try to ignite these chairs, but we wanted a very sort of your minimum input to sort of kick this thing off, to go to actually ignite and burn. So we used a small sort of point seven kilowatt Bunsen burner underneath the chair in the same location. And so before we go into the day too much, I should also give a shout out to the students who helped me out with that. I want to give a shout out to Johnny Reep, who is a fellow PhD student at the University of Edinburgh and my friend Ian Oshway, who were both essential in helping me run all these experiments, because 25 repeats hood scale experiments is not a one man job. Imagine there, yeah, and so the results. For anyone who wants to see this, we recently published a paper in fire technology. Now, obviously, the results are a little more complex than just looking at a scatter plot of time to ignition data for this stuff, and so the degree of complexity was here to the end of the degree now. Now we're looking at time resolved transient burning rates, and so one question you have to ask is okay, what does the heat release rate of a complex fuel package even really represent? There are variations. What's causing that? The heat release rate that you're seeing is some combination of the rate at which the flame is spreading over the fuel package, the rate at which it's actually burning in situ, what is the heat of combustion, of the resulting pyrolysis gases.
Speaker 1:All of these sort of different parameters play a role in the heat release rate To clear out one thing it was a chair put under a hood or was it like a room corner apparatus?
Speaker 2:What was the setup? That's a great point of clarification. So we were just burning these in an open furniture calorimeter.
Speaker 1:So literally, you're in an open space, no feedback.
Speaker 2:Something we actually changed later is we actually did run some experiments in a room corner with the same chair, and I can get to that.
Speaker 1:Cliff hunger too. Okay, I can get to that too.
Speaker 2:We can go back to that at the end. But yeah, so these chairs obviously show a highly transient behavior. So each one shows this very clear. Once you ignite it, there's a clear growth rate, there's a growth through a peak heat release rate, there's a decay rate and eventually the chair burns out. And there's one figure in that paper that I love which is just all 25 heat release recurs, just smacked on top of each other. I think you might remember seeing it in SFP Berlin. It's what I've been referring to as our spaghetti plot. It's just all the curves on top of each other, and so you end up with that. If you look at that, you end up with a substantial degree of fluctuation between the trials and if you sort of take any case, you can sort of see each individual curves going up and down throughout the plot. But if you take that sort of central region of the data, you have a peak heat release rate around 300 kilowatts or something like that. But if you take that central region at any given time, you have a scatter of almost 200 kilowatts in time, right? So the fluctuation is, if you're looking on a time basis, is very large, and I think one of the most important observations, if there's anything to take away from these experiments, is that if you look at this on a time basis, taking an average in time is kind of misleading, right, because if we any one of these individual curves, you can sort of see this sort of general trend of an increase to a peak heat release rate, a decay, and there you know, it sort of makes sense when you look at curves individually and if you take two individual curves you can start to see similar trends in those behaviors. But if you take an average in time, then all of a sudden you get the output no longer represents any one of the individual input curves, right, and there's a very distinct reason for that. That's sort of one thing to take out of this is, if you're looking at these complex fuel packages, these very realistic fuel packages, like I can think of at least it does, and you must be able to of studies where people are taking complex fuels, like car fires, right, compartment fires, and if you're drawing an average through these, it's something to sort of keep in mind that there's a degree of sort of complexity in the transients of these.
Speaker 1:To give the listeners some reference frame, however, I highly recommend reading the fire technology paper or at least skimming through the fire technology paper. The link is in the show notes and it's on open access so everyone can access it and it's really great paper. It's worth to just go to like figure three three and look at the heat release plots. The thing is that some of those courses of the fire you've got would be fitting to more or less medium growth curve. Some of them would be closer to a slow growth curve. I mean the peak heat release you like. I'm not sure if it's difficult to get the close, of course, but the peak heat release rate ends up at like 200 ish kilowatts. But it can happen as well as in like third minute of your test, as much as in like 10th minute of your test, right, and we are applying this to a transient problem of human evacuation in the building. I've now made the jump in my mind to practice. As an engineer, I would take a curve like this, I would put it on this into my CFT and I would say, okay, in this case, you know, my occupants have seven minutes to escape. And the other person says okay, I've burned the same chair, and in my case they have three. And who's right? Of course the guy with the seven minutes wins, because it's this design works and the other guy's design is magically incorrect. Right? And they are conducting the exact same experiment in the same exact apparatus with exact same material ignition and everything. That's just crazy.
Speaker 2:And I think one thing that's really important on the note is talk about yes, we have these variations and, like you said, you can go anywhere from a medium to slow growth in these curves. But why is that the case? I think that's something that we really spent a lot of time articulating in both the paper and presentation senses. But what's really important is if you look at these experiments, they look very different on a time basis, but each one of them follows something like seven key events that occur in every single experiment, and if you identify those events, then all of a sudden you can contextualize the heat release rate. So I mean, I won't go into all of them in detail, but I'll just pick a few, for example, that I list them, I see the plot in front of my eyes.
Speaker 1:So there's a phase of horizontal spread, a phase of upward spread, there's a phase of in-depth burning foam burnout then a mechanical collapse of a chair, and then you enter a long smouldering phase. So it's kind of, if you look at the chair, it's like you're setting up the fire to the bottoms. Eventually the fire spreads to the rest part of the chair, then it burns completely, and then eventually, different stages of at which the fuel runs away. So now, going to your favorite phases, that's a perfect summary right.
Speaker 2:So you notice all of these things happening in all of the trials. Every single trial follows this. They all occur at different times. So if you go from that sort of horizontal spread where the ignition ignites the seat cushion and then that seat cushion spreads to the backrest, that time period is characteristic of a certain kind of fire growth and then the time in which the flames go up the backrest is characteristic of a certain kind of fire growth On a time basis. They're all occurring at different times. But if you actually align instead of aligning the data to the time, you start aligning it to these events. So line up all the curves so that they're in the same, in sync, when the backrest ignites or when the backrest collapse at the end, then all of a sudden you get a curve, that in average curve that actually represents the data that you get. So that's again in more detail in the paper. By using these events you create an average that actually represents each of the individual trials instead of sort of a smear across all the time result data.
Speaker 1:And then you apply some, because I also see the time is uncertain in this plot, so you're not saying me explicitly when the spread changes from horizontal to upward 192 plus minus 90.
Speaker 2:Yeah, exactly yeah.
Speaker 1:Eventually you have to pick up some average transition time and apply that to your curve, more or less.
Speaker 2:True, but I mean, the interesting bit of presenting the data that way is that you can effectively probabilistically recreate these events right, because the if you sort of appreciate that the events drive the heat release rate, then we can do is if I can predict when these events occur. I can start to predict the behaviors in the heat release rate plus or minus some degree of error, right, and so that's something that we haven't done. Yes, copious amounts will work on yet, but this idea that you could probabilistically recreate complex fuel packages gives us a you know, that's a new input that you could use, is perhaps a design fire type scenario. Instead of starting to say that I'm gonna take any one of these individual curves, I can say, okay, now I at least know what's driving the process Right, and so if you can appreciate that, then all of a sudden you can start to like hell for things that you haven't yet observed in your experience.
Speaker 1:So once you go from just viewing it as a time-based Problem, so plotting heat release rate versus time, interviewing it now as a phase-based problem, is done. Certainly in the heat release rate less. Let's start with that. Are you capable of grasping it better? Yes, that's a great question, absolutely.
Speaker 2:So what we saw is, once we started using this sort of event-based system, our ability to predict the uncertainty on a heat release rates went down Dramatically. Now you have to add a pretty tight error bar on your heat release rate at any given event Relative instead of time and so, of course, we're doing all this for this one very specific Upholstered chair that we happen to you know procure for these experiments. But this idea can be applied to any complex experiment, right? So if we're looking at compartment fires or if we're looking at anything else where you know Stochastic variations are causing fluctuations in our results, by just simply pointing a camera at it, being like oh yeah, well, of course, because at this time this transition always occurred really helps contextualize a lot of you know these variations that we see. So quantifying what's my uncertainty on my heat release rate becomes a question of less about what's my scatter in time, what is my certainty predicting this fire behavior.
Speaker 1:I love this because you're touching something that I have intuitively felt for a long time I think we've even discussed this with my speed point on the car park episode that that cars burn in phases. It's. It's not a thing you set a fire to and you get an outcome. And for me there was always this critical events in the vehicle fires like either the windows broke or the fuel tank has collapsed. If you look at any Heat release rate curve of a vehicle fire, I would say with the large certainty I'm not sure if it's a proper word in this episode, but I think you can be quite certain that if you see a peak heat release rate on that plot, it's either the windows broke or the fuel tank broke and then and some fuel is released, whatever the fuel is of that car and we're now also making a paper on that with with my student Bartosz and it's something once we started going one by one on those experiments is something that we can confirm that it's.
Speaker 2:It is like this indeed, and also I should also give a shout out to those missed experiments that we talked about. I mean they noticed was the you know, the giant, the acceleration to this giant heat release rate for their upholstered Furniture fires occurred when the bottom barrier of their fabric cushion failed right, which they created a giant fire. So I mean other people are also, you know, are recognizing this, and I think that is a very powerful thing to say. These key Observable events, then drive this process right.
Speaker 1:So the practical take out of that is actually that you can now create quite a reliable design fire, to be honest, because if you care less about how quickly stuff can happen, you can focus on how big the fire in its different phases could be, you know.
Speaker 2:But this is, this is a really interesting Transition to sort of one of the questions you asked earlier, which was was our experiment in an open hood or was it in a corner? Yeah, okay, I mean we were able to sort of. I would once I don't want to mitigate we were able to pretty reasonably characterize the uncertainty for this particular fuel package in this scenario. So the the leap that you have to make when you're saying, but I know now I'm gonna use this heat release rate to then be my design fire Is that you have to assume that the fire that's gonna occur in your space is the exact Fire, is following the exact events in the exact same scenario. Now, in some cases that might be a reasonable assumption, right, not a single burning item in a compartment With minimal interactions with other items around it can probably be reasonably approximated under furniture calorimetry. But what happens to I think you mentioned this earlier if you put it in a room corner, or what happens involves now you're in the corner of a room, or what? What if I ignite it in a different location? What if I, you know, put a throw pillow on it? Right, yeah, there's always different flexes you have to start thinking about for your actual fire that you're modeling. So we did a series of experiments that we briefly talked about in an SFPE Extra article that was online, where we put a few of these in a room court, like a corner configuration made of gypsum wallboards, and we looked at the heat release rate differences. And what was really interesting is we noticed that because if you read in the literature, we know that if you put something in a room, or two, or a corner configuration, you're definitely gonna increase your flame heights. You're gonna, you know, there's gonna be less entrainment reaching it. So you're getting to affect the temperature distribution in your plume and some sources might suggest that it increases your heat release rate by factor of four. Some sources will say by factor of four exactly. But what's really interesting it's all about in terms of affecting your heat release rate. It's all about what are the events and the drivers that are then, you know, driving this heat release rate process right. So there are some scenarios in which, if you have that you know, increased flame height, you have, you know, different degrees of radiation out to your fuel surface that might drive the process differently. But for our scenario, what we found was the heat release rates for all of these wall corner Configuration type experiments fell within the scatter of our baseline configuration, so the heat release rate wasn't all that different. You got slightly faster growth rates and things like that. But dude, but effectively, if you're looking at this as a, you know, a like-for-like comparison they fell within the uncertainty margin of the baseline. But we also ran a bunch of experiments with heat flux gauges pointed at the chairs, right. Okay, what's really interesting is we noticed that there was a Substantially larger heat flux, though in the wall corner configuration. And so you from which makes sense, right, because you have taller flame heights. You now have a gypsum wall that's heating up, so that's gonna radiate to some degree, but from a, so it. But that's cool experimentally, but thinking from an engineer's perspectives, okay, so not. I can't always just say that my heat release rates gonna increase in the corner, but something that you might be able to say is well, we are definitely gonna get more radiation from this, though, right, because taking that chair into a wall corner configuration took it from like five to, you know, maybe ten kilowatts per meter, squared about a meter away, to 15 plus, right? So now you're pyrolyzing fuel, now there's a potential for a secondary ignition, and so that's a really interesting sort of step change, you know, in a design firetight process but again connecting it to your PMMA studies, you're in an inherently reducing the send the because you're exposing it to higher heat flux.
Speaker 1:Right, although PMMA was ignition time to ignition, here's a burning grade but but I guess mechanisms could be the same you eventually reach High enough heat flux where you just consume the entire thing at once and then you very quickly Move from the spread the phases into burning in depth phase where you have the peak right.
Speaker 2:Yeah, absolutely, but it's an interesting sort of difference there and and we saw also not to go not to go into too many more details but you know we saw very different results if you ignite the chair in a different location, for example. Right, okay, yeah, and certain locations will get much faster Grows to peaky release rate, which I mean it's. That's, for example, if your growth rate is sort of the only part you care about and a lot of things, like you know, sprinkler calculations, like I used to do when I was, you know, if I was, like you know, working as an intern fire engineering firms over in the US, if we're running sprinkler calcs or deep tech models, all we cared about normally was the growth rate, right, and that gave you your time to your sprinkler activation. But we noticed the growth rates were way faster if you ignite it in certain locations versus others, right. So I guess, as engineers, how do we deal with that? How do we know that the ignition source that's gonna match our Signfire scenario is represented by the experimental data we're using?
Speaker 1:but there must be some Repetible. Like you said, the science was the same, so I assume the peak heat release rate Eventually was a function of the entire object burning at its maximum capacity, whatever that is. So that sounds like something not that much time reliant to me. So have you observed like the peak heat release rate overall being like certain to a degree?
Speaker 2:That's something that we've been looking into and that's a question that we would like to answer, because the idea of I guess in first order to answer that you have to think about what is the peak heat release rate, and that's something that's a lot of time scratching your heads about. Is there a physical basis for what the peak heat release rate should be? Right, because if you sort of do a thought experiment, let's take a couch first If you ignite one side of the couch and you have horizontal flame spread all the way across that couch, as the flame is spreading you're also burning within, you know, in the depth of the couch. So flame spread is slow enough to go from one side of the couch to the other. There is a potential you'll start burning out the other side of the couch. Now, if you're in a room environment and you have like a smoke layer and all of a sudden now you're preheating the couch a little bit, you might get faster rates of flame spread, which means you have the entire couch involved. But between scenario A, in which you have burnout, and then the flame still spreading, versus the entire couch burning, you're going to have a very different peak heat release rate. Right, because the peak heat release rate is this complex intersection between your mass burning rate, the area of the item that's flaming, and this idea of like what's your effective heat combustion. So we noticed some similarities because for a lot of these scenarios the progression was the same we ignited the seat cushion, then the seat cushion was still burning by the time that the backrest ignited and it all started burning out around the same time. But that's not always like a guaranteed condition. So the peak heat release rates that we saw were all actually pretty reasonably, you know, within I don't know, something like 50 kilowatts or something to that effect. We talked about a little bit more in the paper. But this idea that has to be paired with this idea of well, is a peak heat release rate an intrinsic property of the fuel package? And I think the answer is no right. It depends on scenarios in which it's burning.
Speaker 1:There's also the aspect of chemical composition, as your materials are exposed to radiation and then to the combustion themselves. As you said, the heat penetrates into the depth of the solid material and, as we know from gravimetric experiments, there are those peak releases of gases and stuff. Eventually you are past the peak and it's not as intense. So I guess that the theoretical peak heat release rate would be if you could capture the moment in your timeline where you get, you know, the most of your material into the peak mass loss from the TGA. You know if you could create those conditions where everything reaches its peak, of generating volatiles at the same time in the most unified manner. Every material in your chair reaches their peak TGA the same moment that you would have the 30. And it couldn't go any higher, like the chemistry would not allow you to go any higher. Of course, in reality there are many transients to that One the frame spread that you said, the heat flux, the feedback loops, even how long it was pyrolyzing again, because perhaps it was not pyrolyzing at its highest speed but already has pyrolyzed all the volatile matter that was there to combust Like. Think about exposed timber that eventually charred off and now you have a solid material, char layer and no more gases from it. That is the ignited set. Perhaps if you somehow recreated those critical conditions in which it gasifies the quickest, you could get some crazy peak heat release rates. But then again, to what extent this it would be an artificial construct, like it would not represent reality, because in reality you'd never reach those conditions.
Speaker 2:And I mean that's a really interesting point and I think that one thing that we that was actually a natural progression from that is the conversation you had with Lucas Arnold not that long ago and talking about if modelers, for example, are trying to match an experimental condition, it depends on which one they're trying to match. So are they trying to match the couch in which you've already burnt out half the couch and the flame is still spreading, or is it the case in which you actually have cost of flame spread? And what are the physical processes that dictate whether or not those two different scenarios occur? And are the experimentalists quantifying those things actually in an agreement with the modelers? And that becomes really interesting sort of discussions that you see at the MacFP workshop, for example through IFCZ. But I think that's a really interesting industry problem. Right At the end of the day, we have to figure out all these intricacies.
Speaker 1:Now using my brain of an engineer, if I had to create the design fire from your concept of phases and your observations, I would simply like I would simplify your phases into phases of growth and phases of, like, steady burning, and I would honestly just take the averages of the heat release rate values but apply them to the minimum times you got. So I would take the average fire but the the soonest I got, though I'm probably destroying the total heat release rate with this approach. Like you, have to forget about physics sometimes in fire engineering, but I think that would give me a reasonable design fire because I would capture the intensity as in some way representative intensity of the of the fire, and I would capture the hazard related to the time, because the time, if we consider fire as a time phenomenon and transient phenomenon from all views, like evacuation, growth, toxicity, etc. I think using the quickest fires like this is the worst case scenario. You know it's if your system works on that, if the fire grows slower than you're capturing that as well.
Speaker 2:This is the thing that we've been sort of spinning our wheels on, thinking about quite a bit, because it's this is the mean I ask every time we present this work. I like to ask the audience, I show them the spaghetti plot, I'm like all right, so what do you choose? What's your design fire that you're taking from this? Because, I mean, my engineering brain also goes to the reality that you could envelope the whole curse. Right, you could say I'm taking the absolute extreme. Forget the total heat release. I know I'm breaking physics, but like that's the most quote unquote conservative way to go. But then you have to start asking the question of why am I even using real data? Am I trying to match a realistic sort of condition based on physics? Or am I trying to create some sort of artificial engineering solution which you know, the alpha t squared type fire curves which are based on, you know, growth rates based on real experimental data? But you are, you are losing the precision for the sake of a sort of more general analysis, and so it's an issue. So, when you're looking at these data, what do you do? And I think it also but a really important thing to consider before saying this is the curve I'm going to take is saying the reason that we separated, like the growth phase into two different curves, for example, is because there's two different physical mechanisms driving that heat release rate Right. So early space is horizontal spread and for the later phases, upward spread. Both of those are in completely different timescales and grow a completely different rates. So if you want to drive an alpha t squared curve through that, you can do so, but you have to do so knowing that you're ignoring two different competing phenomenon, which is OK if that's, if you acknowledge that, so to speak, because you can't count for every complexity. But that's one way to sort of slice it. The alpha t squared framework is largely based on something like a really growing fire anyway. So alpha t squared for the first half sounds pretty good, but then maybe you start looking more like an exponential spread for the upward spread rate. So now all of a sudden, you're doing so, eyes wide open, to linking these to physical mechanisms driving the problem.
Speaker 1:I've already cleaned in the podcast that for me design fires are not representation of real fires and I don't think they ever been. It's more like a test that I apply to my model, like C of D model of a building, C of D model of a compartment. That's my model. Design fire is my test and I never thought this being a representation of a real fire. What's a real fire in the building? But for me, if I do a hundred shops and each of them I apply to an a half megawatt design fire, you know I have a pretty good overall idea of how conditions in this shops vary in this two and a half megawatt fire and based on that I can do my engineering assumptions. And this is the type of fire engineering that I'm very comfortable with, even if my design fire is completely artificial. But if you go into fire engineering where you would take one random curve from one random paper that just fits your agenda and you put that into your CFD model, where you have completely changed the domain, the feedbacks, the environment in which the fire is placed, and you say this is the outcome of what would happen if a real fire, real car, burned in my real car park, this is the representation of reality. This is fire engineering that I am very unhappy with Because, like my mentor, it's something that really stuck with me. My mentor, Professor Czernecki, once asked me do you want to be roughly correct or precisely wrong? And I'm, like it's like this is the same case. Like, do I prefer to apply a design fire and artificial concepts into my car park and just have the outcome I understand and can interpret? Or I want to put a very precise piece of measurement that completely does not make sense in this setting, Then base my judgment on something I don't even trust it being real. Like, I immediately understand that the moment I took this curve from laboratory and put it into my building, I've already made the decision to forget about physics. Right, this is a challenging question for fire safety engineering what design fire really is?
Speaker 2:Yeah, absolutely. The hard part is we still need to do our jobs as fire engineers. We still need to be able to do the analysis and to put fire safety systems through the paces. On the flip side of that, we also are in a field of safety. Our margins of errors have substantial consequences to people, property and the environment If things go wrong. Right Counting for some degree of variability I think is important. If nothing else is taken from this discussion, I guess is the idea of if your inputs are based upon real data or even just sort of a rule of thumb that people are taking, Appreciating the idea that actually we haven't done enough to show that that is right all the time right, and understanding the times at which this fluctuations might actually alter the outputs of your analysis is kind of a critical thing to consider for the sake of designing for safety. Because I mean, yes, you're right, we can do all these amazing probabilistic recreations and do all this cool stuff with the data from our chairs, but is that actually going to be the way to develop the design fires? Maybe there is a way through which that becomes a way to do reliable design fires, but the concept of appreciating what you don't know about the data that goes into these design fires is something that I think every person can at least take away and sort of keep their back their head.
Speaker 1:Fantastic. We could conclude the episode in here, but there's still a one cliffhanger to be resolved the total heat release rate. So was that certain?
Speaker 2:So there's two things that we looked at. We looked at the total heat release in the CO yield. Those are two things that I was hoping to look at and, like you know because like looking back in the cone data, you know, plucking 100 trials of time to the mission, you can run some pretty simple statistics on it I wanted something to pull from you like all right, let's pull yields and let's pull a total heat release, right, and the total heat release was, as you would have guessed, extremely reliable. So we were able to say, you know, it was within these error bars and, if anything, it actually correlated really well to the effective heat of combustion times, the mass of the whole thing, foam in the chair. We were able to show that there was a physical basis for that total heat release. So it turns out, even through all these complexities and all that stuff, the time result, heat release rate, the total heat release actually did come back sort of as a consistency that you can kind of see with like the time to ignition data for PMA. But what was interesting is we noticed something sort of of the opposite effect for the CO yield. So I would encourage people to look at the paper to sort of see this more deep. But if you actually look at the time resolved yield of CO over the course of the experiment, you'll actually notice that it's highly transient, right. So in the beginning you have quite a large CO yield and then you have a period in which you have a very low CO yield and then you have a period at the end again where the CO yields are kind of off the charts. And what's really interesting is this looks a bit daunting when you first look at it because you're like, oh my gosh, turns out if you were to pick a CO yield from the back of the SFB handbook, you know if you're talking foam. You're sort of at the lower limit of anything that we measured in the whole experiment, right. So to take a constant yield would really underestimate the total CO generation. And it makes sense when you think about it, because in the beginning you have tons of pyrolysis gases and not much flaming going on, right. So if you can imagine this chair, I put the pilot underneath it. In the early phases the heat release rate is growing slowly, but there's lots of areas which are undergoing pyrolysis and they're giving off gases, but those aren't burning and what we know from sort of combustion chemistry, is these pyrolysis gases have a lot of carbon monoxide, lots of CO in them, right. But once those gases ignite, the flame sheet provides the sort of you know this sufficient temperatures for which the CO can be oxidized into CO2, right. So then our CO yield goes down substantially during flaming. But then, as the flame sheet starts to break down towards the end of the experiment, your CO yields start to creep back up. So, in terms of modeling applications, of trying to understand the you know, how do I apply a CO yield? If you're looking at real fuel packages undergo these phases, then understanding the phases are really important in sort of being able to accurately model what is my CO generation. And that was sort of the, while the total heat release was a nice sort of closed like bow on top of everything, the CO opened up all sorts of questions. That requires some further research.
Speaker 1:I'm not sure why I'm saying total heat release rate. I just heat release rate is so embedded in my head I simply cannot say heat release without adding great at the end. And you know what's driving me crazy that I know so many people that would just take your conclusions in here and apply at the same time the maximum burning rate and the maximum CO generation, because that's conservative, you know, ignoring that there's a very physical distinction between version one, where your pyrolysis gaseous burn, and version two where they don't, having leading to completely two separate pathways of outcome. I know people, a lot of people, who just merge them. Conservative. Let's do this. Anyway, david, fantastic fire science. Like it's really exciting how much you can do with simple experiments, simple strive for creating the world's largest fire and stuff like that, the most complex settings. And I know more and more people who find the beauty in really understanding the simple mechanisms in fire, simple access, like artificial concept, because we all know that when you go in depth into fire science, the deeper you go, the harder it gets. So I really appreciate this, this kind of science, and I hope to talk to you soon again in the fire science show. Thanks for coming, absolutely.
Speaker 2:No, thank you so much for having me. This has been a wonderful chat. Thank you again.
Speaker 1:And that's it. I hope you've enjoyed it. I hope I did not overhype this. For me it was a really great conversation and really important episode of the fire science show. You know I've done my trials on design fire episodes and I hope they to some extent are in line with what David was presenting us in this discussion. David has a lot of firsthand experience in testing those fires and measuring those fires and observing them and figuring out where the discrepancies and ascendies come into those fires. So I'm quite happy that my observations are in line with what he's explaining. Of course I didn't go that deep into this. Kudos to David for his hard work. David has also asked me to give a shout out to Professor Glern Torncroft, who is the professor who put him into the resistor lab that he mentioned as a story in the episode, and actually Professor Torncroft is also a co-author of his PMMA paper. That's really nice that it sparked the interest in measuring uncertainty and went so far that they've written a paper together on the properties of PMMA Fantastic, I love this story in a way. Back to the practical takes from this podcast episode Gosh, there are so many. First of all, the phases of fire. How, by slicing the fire into phases. You can distinguish them independently and figure out some statistical properties of each of the phases. This is a really far reaching conclusion that we can really put into practice if we get more data on common fires. This is the future guys. This is how we're going to do our design fires in the future. Another thing I did not really capture that and follow in the podcast episode itself. It really struck me when I was editing the podcast that David mentioned the ability to use this knowledge for probabilistic fire definition, and this is a beautiful concept. This could really work out because if you know the phases, if you know the probability of transitioning from phase to phase, you could really go on with very good probabilistic design fires. So I love it and I'm looking forward for more work from Edinburgh and David's team. I'm crossing fingers for his PhD and I am looking forward for all the fire science he's going to put out once he is a doctor at Edinburgh. A lot of possibilities and I'm really keen to work with them on topics like this in the future. So that's it for this episode. I am overly excited. I'm sorry, apologies, but I love fire science. I love this. This is the exact thing. That is my passion and you can hear it. Anyways, next week, another interesting topic in the world of fire science, another, hopefully exciting for you subject. So yeah, let's meet again next Wednesday. Same place, same time. Cheers. Bye, this was the fire science show. Thank you for listening and see you soon.